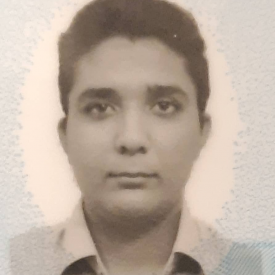
معین محمدزاده فردفینی
پیش بینی تشریحی قیمت سهام با استفاده از اطلاعات چند منبعی برای طراحی سبد بهینه در بازار سرمایه
- دانشجو
- معین محمدزاده فردفینی
- استاد راهنما
- ایمان رئیسی وانانی
- استاد مشاور
- محمدتقی تقوی فرد
- استاد داور
- پیام حنفی زاده
- مقطع تحصیلی
- کارشناسی ارشد
- تاریخ دفاع
- ۳۱ شهریور ۱۴۰۳
- ساعت دفاع
- چکیده
- پیشبینی قیمت سهام یکی از چالشهای کلیدی در حوزه مالی و سرمایهگذاری است که به دلیل نوسانات غیرقابل پیشبینی بازار و تأثیر عوامل متنوع اقتصادی، سیاسی و اجتماعی، اهمیت فراوانی دارد. با افزایش حجم دادههای موجود و تنوع اطلاعات، استفاده از مدلهای پیشرفته برای تحلیل این دادهها و شناسایی الگوهای مؤثر بر قیمتها میتواند به بهبود تصمیمگیریهای سرمایهگذاران کمک شایانی کند. هدف این پژوهش، بررسی تأثیر دادههای چندمنبعی و مدلهای یادگیری عمیق بر پیشبینی قیمت سهام و طراحی سبد بهینه سرمایهگذاری است. دادهها از منابع مختلفی شامل پایگاههای داده مالی، اخبار مالی آنلاین و تحلیلهای تکنیکال استخراج شدند. این پژوهش در سه سناریوی مختلف انجام گرفت: سناریو اول، پیشبینی قیمت سهام صرفاً با استفاده از دادههای خام قیمتی صورت گرفت؛ سناریو دوم، پیشبینی با ترکیب دادههای قیمتی و اندیکاتورهای تکنیکال انجام شد؛ و سناریو سوم، پیشبینی با استفاده از دادههای خام قیمت، اندیکاتورهای تکنیکال و تحلیل قطبیت اخبار مالی صورت گرفت. نتایج این تحقیق نشان داد که ترکیب دادههای چندمنبعی بهطور قابلملاحظهای دقت پیشبینی قیمت سهام را افزایش داد و مدلهای یادگیری عمیق، بهویژه BiRNN و BiLSTM، بهترین عملکرد را در این زمینه ارائه کردند. همچنین، ابزارهای هوش مصنوعی تفسیرپذیر مانند SHAP و LIME به شناسایی عواملی که بیشترین تأثیر را بر تغییرات قیمت دارند، کمک کردند و امکان درک روابط پیچیده میان ویژگیهای ورودی و نتایج پیشبینی را فراهم آوردند. در نهایت، با استفاده از نظریه مارکویتز و نسبت شارپ، سبد بهینه سرمایهگذاری طراحی شد که بازده سالانه مورد انتظار ۶۰.۳۹% و ریسک ۱۵.۱۳% را بهدست آورد. این پژوهش بهعنوان یک گام کلیدی در ارتقاء فرآیندهای پیشبینی قیمت سهام و بهینهسازی سبد سرمایهگذاری شناخته میشود و میتواند مبنای مناسبی برای تحقیقات آتی در این حوزه باشد. با توجه به نتایج بهدستآمده، اهمیت بهکارگیری رویکردهای نوین مبتنی بر هوش مصنوعی و دادههای چندمنبعی در تصمیمگیریهای سرمایهگذاری و مدیریت ریسک تأکید میشود.
- Abstract
-
Stock price forecasting is one of the key challenges in the financial and investment field, which is very important due to the unpredictable fluctuations of the market and the influence of various economic, political and social factors. With the increase in the amount of available data and the variety of information, the use of advanced models to analyze these data and identify patterns affecting prices can help improve investors' decisions. The purpose of this research is to investigate the effect of multi-source data and deep learning models on stock price prediction and optimal investment portfolio design. Data were extracted from various sources including financial databases, online financial news and technical analysis. This research was carried out in three different scenarios: the first scenario, stock price prediction was done using raw price data only; The second scenario, forecasting was done by combining price data and technical indicators; And the third scenario, the prediction was made using raw price data, technical indicators and polarity analysis of financial news. The results of this research showed that the combination of multi-source data significantly increased the accuracy of stock price prediction, and deep learning models, especially BiRNN and BiLSTM, provided the best performance in this field. Also, interpretable artificial intelligence tools such as SHAP and LIME helped to identify the factors that have the greatest impact on price changes and made it possible to understand the complex relationships between input characteristics and forecasting results. Finally, using the Markowitz theory and Sharpe ratio, the optimal investment portfolio was designed, which obtained the expected annual return of ۶۰.۳۹% and the risk of ۱۵.۱۳%. This research is known as a key step in improving the processes of stock price forecasting and investment portfolio optimization, and it can be a good basis for future research in this field. According to the obtained results, the importance of using new approaches based on artificial intelligence and multi-source data in investment decisions and risk management is emphasized.