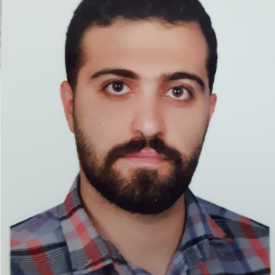
مهرشاد متانی
پیش بینی روند قیمت سهام بورس اوراق بهادار تهران با استفاده از مدل مداری مبتنی بر گراف پیچشی و شبکه عصبی پیچشی
- دانشجو
- مهرشاد متانی
- استاد راهنما
- میثم امیری
- استاد مشاور
- محمد مهدی بحرالعلوم
- استاد داور
- مسلم پیمانی فروشانی
- مقطع تحصیلی
- کارشناسی ارشد
- تاریخ دفاع
- ۲۰ تیر ۱۴۰۳
- ساعت دفاع
- چکیده
-
چکیده
پیشبینی روند سهام به علت پویایی و پیچیدگی های خاص خود، یکی از چالش برانگیزترین موضوعاتی بوده است که در راستای آن، محققان انواع مدل های یادگیری ماشین و یادگیری عمیق را توسعه دادهاند. در پی این مطالعات عمدتا تاثیر دیگر سهام بر پیشبینی روند قیمتی در نظر نگرفته شده است بنابراین در این پژوهش به دنبال بررسی عملکرد مدل مداری مبتنی بر گراف پیچشی و شبکه عصبی پیچشی بوده و برای پیشبینی روند سهام از روش شبکه گراف پیچشی مبتنی بر ویژگی شبکه عصبی پیچشی استفاده شده است، که در آن هم اطلاعات بازار سهام و هم اطلاعات سهم هدف در نظر گرفته شده است. سپس این داده ها به تصویر تبدیل شده و با استفاده از شبکه عصبی پیچشی دوگانه[۱] تجزیه و تحلیل شده و ویژگی های سهام هدف استخراج شد. به صورت خلاصه در این مدل، یک شبکه پیچشی گراف و یک شبکه عصبی پیچشی دوگانه در کنار هم برای پیشبینی روند سهام به کار گرفته شد که میتوانند به طور همزمان ویژگیهای بازار بورس اوراق بهادار تهران و ویژگیهای سهام هدف را ثبت کنند.
جامعه آماری این پژوهش شامل کلیه شرکتهای پذیرفته شده در بورس اوراق بهادار تهران در قلمرو زمانی ۷ سال و ۳ ماهه (ابتدای ۱۳۹۶ تا انتهای خرداد ۱۴۰۳) میباشد که سهام هدف از طریق نمونهگیری سیستماتیک از صنایع با بالاترین ارزش معامله و بیشترین نقدشوندگی انتخاب شده است. همچنین، پس از انتخاب سهم هدف، ۳ سهم مرتبط با سهم هدف نیز با توجه به بیشترین همبستگی (رنک-اسپیرمن) از هر صنعت بورسی انتخاب شده است.
نتایج نشان داد که این مدل در شرایط مختلف بازار، به طور کلی عملکرد قابل قبولی داشته و توانسته است بازدهی معقولی را ارائه دهد. در مقیاس نسبی، مدل از ۵ سهم انتخاب شده، توانسته است در تمامی سهام از مدل شبکه عصبی پیچشی عملکرد بهتری از منظر بازدهی داشته باشد، همچنین مدل در مقایسه با شبکه عصبی بازگشتی در ۲ سهم عملکرد بهتری داشته است و در نهایت مدل مورد استفاده در این پژوهش در مقایسه با شبکه عصبی حافظه طولانی کوتاه مدت در ۲ سهم عملکرد بهتری از منظر بازدهی را ثبت کرده است. اما این در حالی است که مدل مداری مبتنی بر گراف پیچشی و شبکه عصبی دارای زمان اجرای بالا و پیچیدگی نسبت به سایر مدل های مورد بررسی میباشد.
- Abstract
-
Abstract
Forecasting stock trends, due to their inherent dynamics and complexities, has been one of the most challenging topics for which researchers have developed various machine learning and deep learning models. These studies have generally not considered the impact of other stocks on price trend prediction. Therefore, this research aims to evaluate the performance of a circuit model based on convolutional graph networks and convolutional neural networks. The proposed method employs a convolutional graph network based on the features of convolutional neural networks to predict stock trends, taking into account both stock market information and the specific target stock information. Subsequently, this data is transformed into images and analyzed using a dual convolutional neural network to extract the target stock's features. In summary, this model employs a combination of a convolutional graph network and a dual convolutional neural network to predict stock trends, capable of simultaneously capturing the features of the Tehran Stock Exchange market and the target stocks.
The statistical population of this research includes all companies listed on the Tehran Stock Exchange over a period of ۷ years and ۳ months (from the beginning of ۲۰۱۷ to the end of June ۲۰۲۴). Target stocks are selected through systematic sampling from industries with the highest trading value and liquidity. Additionally, after selecting the target stocks, ۳ related stocks with the highest correlation (Spearman rank correlation) from each stock industry are chosen.
The results indicated that this model generally performed acceptably under various market conditions and provided reasonable returns. On a relative scale, the model outperformed the convolutional neural network in terms of returns for all ۵ selected stocks. Furthermore, the model demonstrated better performance than the recurrent neural network for ۲ stocks and showed superior performance compared to the long short-term memory network for ۲ stocks in terms of returns. However, it should be noted that the circuit model based on convolutional graph networks and neural networks has a higher execution time and complexity compared to other models under review.