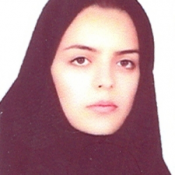
صفورا حسینی
عنوان پایاننامه
پیش بینی خط مبنای انرژی در واحدهای عملیاتی شرکت پالایش نفت شازند جهت ارائه راهکار در بهبود مصرف انرژی
- مقطع تحصیلی
- کارشناسی ارشد
- استاد داور
- مقصود امیری
- دانشجو
- صفورا حسینی
- استاد مشاور
- جهانیار بامداد صوفی
- استاد راهنما
- محمدتقی تقوی فرد
- چکیده
- پیش بینی خط مبنای انرژی در واحدهای عملیاتی شرکت پالایش نفت شازند جهت ارائه راهکار در بهبود مصرف انرژی